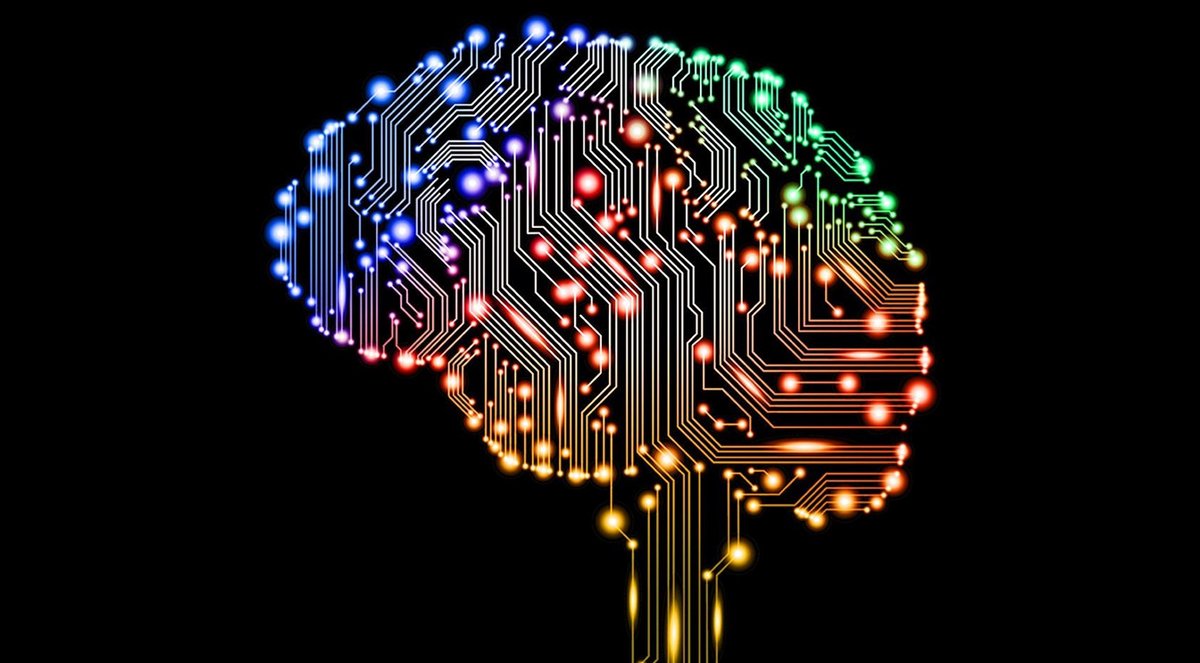
Deep Learning Programming
- 1st exercise has been uploaded!
- 2nd exercise has been uploaded, the submission deadline is 12th May!
- 3rd exercise has been uploaded, deadline is 19th May!
- 4th exercise has been uploaded, deadline is 26th May!
- A company (in the Lake Constance area) is looking for graduate (Bachelor’s or Master’s) with MATLAB / Simulink skills for a full-time position, if you are about to graduate and interested in the position, send me email.
- No exercise session on 22th May!
- 5th exercise has been uploaded, deadline is 2nd June!
- Important date: submitted your project title, group list, and a few sentences description about your project on 9th June
- No exercise session on 5th June!
- 6th and 7th assignments have been uploaded, the deadline is 9th June and 16th June, respectively.
- The presentation schedule has been released!
- Report submission date: 30th August! Late submission will be rejected directly!
Notice:
Presentation schedule
Each group has 35 mins to present their project and 10 mins of Q&A. When you are doing your presentation, you may not finish your model training and performance evaluation, instead you may present your motivation, framework of your approach, data collection, etc.
No. | Topic | Member | Time |
---|---|---|---|
1 | Bitcoin Data (Price, Vol. , Vola) Prediction using historical Data,Twitter Data | Lukas Schulth, Lukas Würtenberger, Henrik Brautmeier | 01.07 |
2 | YOLOV3 network for object recognition | Felix Hinderer, Dimitar Garkov | 01.07 |
3 | Using CNN’s as chess AI | Maximilian Martin Reihn | 03.07 |
4 | Changing images with style of a certain painter | Denis Makarov, Jiaxin Li | 03.07 |
5 | Author Classification of Spooky Literature | Marc Lüttecke, Franziska Weeber | 08.07 |
6 | Using Deep Neural Networks for identifying the authorship of a Twitter post | Adam Norris, Simon Suckut | 08.07 |
7 | Bird Audio Detection challenge | Daniel Metzger, Alexej Gluschkow, Ruoying Li | 10.07 |
8 | Predicting modern art movements using image recognition | Roberto Daniele Cadili, Burak Özturan | 10.07 |
9 | Motorcycle helmet use prediction | Guangan Chen | 15.07 |
10 | Generating rendered ink brush strokes from the raw stroke trajectories | Timon Kilian, Patrick Paetzold, Jonas Körner | 15.07 |
11 | Nowcasting thunderstorms using deep learning on satellite and radar images | Lukas Ringlage, Daniel Färber, Philipp Behrendt | 17.07 |
12 | Recognition of kinship based solely on faces images | Lada Rudnitckaia, Wenxuan Zhang | 17.07 |
13 | Predicting educts of chemical reactions using Sequence-to-Sequence models | Benedikt Bäumle | 17.07 |
Content
This course provides an introduction to deep learning and emphasize deep learning programming. Students will learn about neural network, convolutional neural networks, recurrent neural networks, autoencoders, hyperparameter tuning, regularization, optimization, and more. Theoretical aspects will be detailed throughout the course, but more complicated issues such as proofs of relevant theorems/schemes will not be presented. The contents of the course will be covered by lectures and lab sessions of Python/Keras programming
This practical programming course covers
- Deep neural networks
- Convolutional neural networks
- Hyperparameter tuning, regularization, optimization
- Transfer learning
- Recurrent neural networks
- Autoencoder
Prerequisites
None, if taken as a master course. If taken as an advanced course in the bachelor program:
- python programming
- basic math courses offered in our bachelor programs
- algorithms and data structures
Course works and credits
Credit will be given based on solving assignments and completing a final project. Students are required to use their laptops to solve assignments in lab session. Credit will be given based on two parts: a oral presentation (30%) and a technical report (70%, up to 4 pages with IEEE conference template). The presentation should include motivation, data collection, design of the framework. The technical report should include the framework you implement, analysis of experimental results.
Reference
Textbook
- Ian Goodfellow, Yoshua Bengio, Aaron Courville. Deep learning. MIT press, 2016 Link
Deep learning introduction
- LeCun, Yann, Yoshua Bengio, and Geoffrey Hinton. "Deep learning." nature 521.7553 (2015): 436.
- Lu, Jiwen, Junlin Hu, and Jie Zhou. "Deep Metric Learning for Visual Understanding: An Overview of Recent Advances." IEEE Signal Processing Magazine 34.6 (2017): 76-84.
- Canziani A, Paszke A, Culurciello E. An analysis of deep neural network models for practical applications. arXiv preprint arXiv:1605.07678. 2016 May 24.
Learning
- Adam: Kingma, Diederik P., and Jimmy Ba. "Adam: A method for stochastic optimization." arXiv preprint arXiv:1412.6980(2014).
- Dropout: Srivastava, Nitish, et al. "Dropout: A simple way to prevent neural networks from overfitting." The Journal of Machine Learning Research 15.1 (2014): 1929-1958.
- Batch normalization: Ioffe, Sergey, and Christian Szegedy. "Batch normalization: Accelerating deep network training by reducing internal covariate shift." arXiv preprint arXiv:1502.03167 (2015).
- 1x1 convolution: Lin, Min, Qiang Chen, and Shuicheng Yan. "Network in network." arXiv preprint arXiv:1312.4400 (2013).
- Random Search: Bergstra, James, and Yoshua Bengio. "Random search for hyper-parameter optimization." Journal of Machine Learning Research 13.Feb (2012): 281-305.
State-of-the-art CNNs
- LeNet: LeCun, Yann, et al. "Gradient-based learning applied to document recognition." Proceedings of the IEEE 86.11 (1998): 2278-2324.
- AlexLet: Krizhevsky, Alex, Ilya Sutskever, and Geoffrey E. Hinton. "Imagenet classification with deep convolutional neural networks." Advances in neural information processing systems. 2012.
- VGGNet: Simonyan, Karen, and Andrew Zisserman. "Very deep convolutional networks for large-scale image recognition." arXiv preprint arXiv:1409.1556 (2014).
- GoogLeNet: Szegedy, Christian, et al. "Going deeper with convolutions." Cvpr, 2015.
- ResNet: He, Kaiming, et al. "Deep residual learning for image recognition." Proceedings of the IEEE conference on computer vision and pattern recognition. 2016.
- InceptionResNetV2: Szegedy C, Ioffe S, Vanhoucke V, Alemi AA. Inception-v4, inception-resnet and the impact of residual connections on learning. InThirty-First AAAI Conference on Artificial Intelligence 2017 Feb 12.
Object detection
- DPM: Felzenszwalb, Pedro F., et al. "Object detection with discriminatively trained part-based models." IEEE transactions on pattern analysis and machine intelligence 32.9 (2010): 1627-1645.
- R-CNN: Girshick, Ross, et al. "Rich feature hierarchies for accurate object detection and semantic segmentation." Proceedings of the IEEE conference on computer vision and pattern recognition. 2014.
- Fast R-CNN: Girshick, Ross. "Fast r-cnn." arXiv preprint arXiv:1504.08083(2015).
- Faster R-CNN: Ren, Shaoqing, et al. "Faster r-cnn: Towards real-time object detection with region proposal networks." Advances in neural information processing systems. 2015.
- Mask R-CNN: He, Kaiming, et al. "Mask r-cnn." Computer Vision (ICCV), 2017 IEEE International Conference on. IEEE, 2017.
- YOLO: Redmon, Joseph, et al. "You only look once: Unified, real-time object detection." Proceedings of the IEEE conference on computer vision and pattern recognition. 2016.
- YOLO9000: Redmon, Joseph, and Ali Farhadi. "YOLO9000: better, faster, stronger." arXiv preprint (2017).
- RetinaNet: Lin TY, Goyal P, Girshick R, He K, Dollár P. Focal loss for dense object detection. In IEEE international conference on computer vision 2017 (pp. 2980-2988).
Face recognition
- FaceNet: Schroff, Florian, Dmitry Kalenichenko, and James Philbin. "Facenet: A unified embedding for face recognition and clustering." Proceedings of the IEEE conference on computer vision and pattern recognition. 2015.
Style transfer
- Gatys, Leon A., Alexander S. Ecker, and Matthias Bethge. "Image style transfer using convolutional neural networks." 2016 IEEE Conference on Computer Vision and Pattern Recognition (CVPR)
Generative model
- Variational autoencoder: Kingma, D. P., & Welling, M. (2013). Auto-encoding variational bayes. arXiv preprint arXiv:1312.6114.
- Kingma DP, Mohamed S, Rezende DJ, Welling M. Semi-supervised learning with deep generative models. In Advances in neural information processing systems 2014 (pp. 3581-3589).
- GAN: Goodfellow I, Pouget-Abadie J, Mirza M, Xu B, Warde-Farley D, Ozair S, Courville A, Bengio Y. Generative adversarial nets. In Advances in neural information processing systems 2014 (pp. 2672-2680).
- DCGAN: Radford, Alec, Luke Metz, and Soumith Chintala. "Unsupervised representation learning with deep convolutional generative adversarial networks." arXiv preprint arXiv:1511.06434 (2015).
- Semi-supervised learning: Kingma DP, Mohamed S, Rezende DJ, Welling M. Semi-supervised learning with deep generative models. InAdvances in neural information processing systems 2014 (pp. 3581-3589).
Recurrent neural network:
- LSTM: Hochreiter S, Schmidhuber J. Long short-term memory. Neural computation. 1997 Nov 15;9(8):1735-80.
- GRU: Cho, K., Van Merriënboer, B., Bahdanau, D., & Bengio, Y. (2014). On the properties of neural machine translation: Encoder-decoder approaches. arXiv preprint arXiv:1409.1259.
- Chung J, Gulcehre C, Cho K, Bengio Y. Empirical evaluation of gated recurrent neural networks on sequence modeling. arXiv preprint arXiv:1412.3555. 2014 Dec 11.